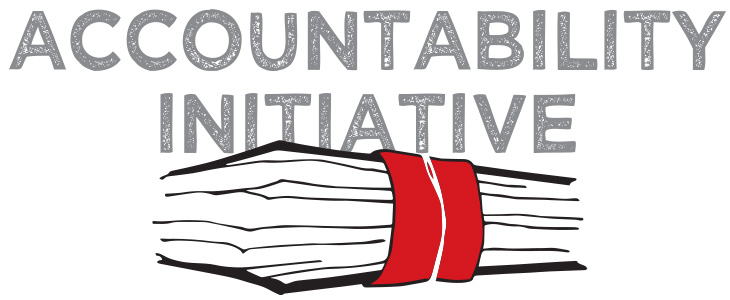
Random- esque
29 March 2011
In the spirit of my previous blog I have endeavoured once again to explain various phenomena, through my own tainted glasses- my home grown typologies. This time around the innocent victim is none other than the statistical technique of randomized evaluations (applause) my fascination for which I can only describe as being random-esque (louder applause please). Positing myself at the very far end of the spectrum when it comes to all things statistical, randomized evaluations was a concept which was entirely new to me. As it would happen, I was recently given an opportunity to attend a workshop organized by J-PAL and ASER Ccentre on understanding the methodology of randomized evaluations. My experience greatly inspired me, which brings me back to why I am launching into a panegyric and going about ascribing my precious little typologies to a statistical methodology.
So let me begin my describing what exactly is meant by the concept. Randomized evaluations constitute one of the methodologies adopted to evaluate the impact of a particular programme/scheme. More specifically the methodology seeks to measure whether programmes or policies are succeeding in achieving their goals. Take for instance a programme like the Janani Suraksha Yojana (JSY) – a conditional cash transfer aimed at reducing maternal and neo-natal mortality by promoting safe institutional delivery among poor pregnant women. Given the large budget of the scheme amounting Rs `1,515 crores (see Accountability Initiative Budget Brief on Health http://www.accountabilityindia.in/article/budget-health/2145-health-sector-goi-2010-11-new), it seems pertinent to assess the effectiveness of the programmes; it’s success in achieving its stated desired goal and objective. Programme effectiveness is usually evaluated by comparing outcomes of those who participated in a programme with those that did not participate in the programme. This is because when we are trying to evaluate how effective a programme has been we are really asking is what would have happened in a situation had the programme not been introduced. In statistics this is referred to as the counterfactual – a hypothetical situation, which cannot be directly observed but can only be mimicked. One way of attempting to mimic the counter factual is to compare those who participated in a programme and those who did not. The key challenge in impact evaluations is to find a group of people who did not participate, but closely resemble the participants. Measuring outcomes in this comparison group is as close as we can get to measuring how participants would have been without the intervention (for more details see http://www.povertyactionlab.org/methodology).
Comparison groups can be constructed in many ways. One of the common methods is to compare the participant group before and then after the programme to measure how the status of the participants changed over time. In the context of the JSY this would imply comparing an outcome such as the number of beneficiaries who accessed institutional delivery before the programme with the number of beneficiaries who accessed such services after the programme. Another comparison group can be those who did not participate in the programme, their status can be compared to those who did participate in the programme. For instance in the case of JSY, it would mean comparing the access of institutional facilities by beneficiaries to those of non beneficiaries. A third way is to constitute those who did not participate in the programme as the comparison and then assess their status both before and then after the programme. Through this method it is possible to measure change in the status of the programme participants (over time) relative to the improvement or change of non participants. In the case of JSY it would imply, measuring the institutional facilities accessed by non beneficiaries both before the programme and the facilities accessed after the programme, compared with the status of beneficiaries. Additionally it is also possible to construct a comparison group of individuals who did not participate in the programme but for whom data was collected both before and after the programme. In this case data is not collected not only on outcome indicators but also other explanatory variables. Impact through this methodology is assessed by comparing those individuals those who participated in the programme and the difference between the two is controlled for. Here again drawing on the example of JSY this would mean considering the status of beneficiaries on a range of outcomes, not only whether they accessed institutional facilities but also indicators such as caste, income and economic status which may influence the access to such facilities.
So if there is a range of ways to create a comparison group then what exactly is the relevance of randomized evaluations? Advocates of randomized evaluations argue that in comparison to all these different methodologies, randomized evaluations do the ‘best job’ in determining the impact of a particular programme. This contention is based on the understanding that the aforementioned methods are based on certain assumption. Thus findings from such survey hold true as long as certain conditions are met with. Take the first method, if we only compare the number of beneficiaries who accessed institutional facilities both before and after the programme then we are implying that the JSY programme was the only factor influencing any change in the access to institutional facilities. This method does not cater to the fact that it may be another variable such as an awareness campaign which might have been more influential than cash transfer in impelling women to access institutional services. The second methodology in comparison is based on the assumption that non beneficiaries are identical to the beneficiaries, except for their participation in the programme. This is a difficult proposition given the wide difference in population. In a similar vein the third method described, wherein the changes in status of the non beneficiaries before and after the programme are compared that with the change in the status of the beneficiaries is employed to evaluate the impact of the programme, relies on the assumption that if the programme had not existed then the two groups would have had identical trajectories over this period. The fourth method which tries to control for variables which may explain the difference in the outcomes of beneficiaries and non beneficiaries, also implicitly relies on the assumption that factors that were excluded because they could not be observed such as religious beliefs which prohibit access to institutional facilities, do not bias the results because they are either uncorrelated with the outcome or do not differ between beneficiaries and non beneficiaries.
Hmm… If your anything like me your probably thinking- ‘if previous methods are all based on certain assumptions which somewhat taint and bias an assessment of a particular programme, then surely randomized evaluations must be riddled with similar problems’. To my great disenchantment my scepticism was discovered to be quiet substantially unfounded. Randomized evaluations I was pressed to recognize represent a more accurate method of constructing a comparison group which closely resembles the participant group/beneficiaries. The methodology adopted in creating this comparison group is termed random assignment. Random assignment involves selecting randomly selected a group from the larger population, say the population of women and then assigning them again randomly to a group that receives the cash transfer under JSY and those who do not received such benefits. The significance of selecting a group of beneficiaries and non beneficiaries from the larger population is that the attributes of those chosen individuals are representative of the entire group from which they are chosen. In other words what is discovered about them is also probably true for the larger group. In statistical terms when a group selected from the larger group is similar to it, they are considered to be statistically equivalent. Further since the beneficiaries and non beneficiaries are also randomly assigned then they two are considered to be statistically equivalent to each other. The group which has been randomly assigned as to receive the programme is then evaluated. Drawing on the example of JSY, this would imply that in the group of women who have been designated as the intervention group would receive cash incentive while the other group of women would not. Since the two groups began from being statistically equivalent, interventions introduced for one group would make it different from the other. Differences seen between them can then be measured and attributed to one having been given cash incentive and the other not. Thus assumptions which somewhat bias the results of the previously mentioned methodologies do not hold true in the case of randomized evaluations.
This of course is only the tip of the proverbial iceberg. Given my limited knowledge I have tried to err on the side of caution by presentation only a small aspect of what this methodology encapsulates. There is a lot more that I am yet to understand, and it would be great if I could get some more insights (this is where you all step in) to make sense of all this random-ness! But for now I am enchanted and random-esque it remains.